Urgent Message: There are no consensus definitions for what defines a “positive” urinalysis in the pediatric population. This secondary analysis of data from a quality improvement project in a network of pediatric urgent care centers used a machine learning approach to determine which variables on urinalysis most strongly predict a subsequently positive urine culture.
Kari Flicker, BS; Jessica Parrott, DNP, CPNP-PC, CNE; Tammy Speerhas, DNP, FNP-C; Turaj Vazifedan, DHSc; Theresa Guins, MD; Jeffrey Bobrowitz, MD; Anne McEvoy, MD; Jade Eves, PA-C; Debra Conrad, MD; Benjamin Klick, MD
Citation: Flicker K, Parrott J, Speerhas T, Vazifedan T, Guins T, Bobrowitz J, McEvoy A, Eves J, Conrad D, Klick B. Development of a Positive Urinalysis Criteria Using a Machine Learning Approach. J Urgent Care Med. 2024; 19(2): 35-43
Model | Description |
---|---|
1. | Completely negative urine: no blood and no LE and no WBCs and negative nitrites and no bacteria |
2. | Any positive values: any blood or any LE or any WBCs, or positive nitrites or any bacteria |
3. | 2011 AAP UTI guidelines: any LE or positive nitrites or at least 5-10 WBCs or any bacteria |
4. | Modified 2011 AAP UTI guidelines: at least moderate LE or positive nitrites or at least 5-10 WBCs or any bacteria |
5. | 2011 AAP UTI guidelines without bacteria: any LE or positive nitrites or at least 5-10 WBCs |
6. | Modified 2011 AAP UTI guidelines without bacteria: at least moderate LE or positive nitrites or at least 5-10 WBCs |
7. | Previous local treatment guidelines without bacteria: at least moderate LE or at least 10-25 WBCs or positive nitrites |
8. | Current local treatment guidelines without bacteria: positive nitrites or (at least moderate LE and at least 10-25 WBCs). |
9. | Previous local treatment guidelines with bacteria: at least moderate LE or at least 10-25 WBCs or positive nitrites or any bacteria |
10. | Current local treatment guidelines with bacteria: positive nitrites or any bacteria or (at least moderate LE and at least 10-25 WBCs). |
11. | Previous local treatment guidelines with hematuria: at least moderate LE or at least 10-25 WBCs or positive nitrites or any blood |
12. | Current local treatment guidelines with hematuria: positive nitrites or any blood or (at least moderate LE and at least 10-25 WBCs) |
AAP: American Academy of Pediatrics; LE: leukocyte esterase; UTI: urinary tract infection; WBCs: white blood cells
Abstract
Background: Urinary tract infections (UTIs) are a commonly encountered diagnosis at pediatric urgent care (UC) centers. The urinalysis (UA) is usually the initial study in UC settings used to guide decisions regarding initiating empiric antibiotics and/or pursuing urine culture. However, studies in pediatric UC settings examining the ideal threshold for a positive result are lacking.
Methods: UA result data were extracted from the records of 6,327 pediatric patients, which were collected as part of a previous QI project. Logistic regression was used to determine the predictors of positive urine cultures. Decision trees for a positive UA result for both clean catch and catheterized specimens were created, and test performance and characteristics were assessed.
Results: The presence of a positive nitrite result was found to be a strong predictor for a positive urine culture. For nitrite negative in specimens obtained by catheterization, the presence of leukocyte esterase (LE) and ≥ to 5 white blood cells per high powered field (WBC/HPF) had the greatest accuracy. For clean catch specimens, the presence of at least moderate LE was the best predictor.
Conclusion: Using a machine learning approach, criteria for a positive urinalysis were developed for the pediatric UC setting.
Introduction
Urinary tract infections (UTIs) are common across pediatric populations. While pediatric UC specific data is lacking, the overall incidence of UTIs is 1.5% in children under 2 years old[1] and 6% in females under 6 years old.[2]
Urinalysis (UA), among the first laboratory tests used in medicine, remains a widely available, low cost clinical lab test with broad applicability.[3] UA may consist of chemical examination (eg, colorimetric dipstick) and/or microscopic evaluation.[4] Among the data provided by UA, surrogate markers of infection are evaluated to determine if antibiotics are indicated while awaiting urine culture results.4 However, the exact definition of what constitutes a “positive” UA varies across studies. The American Academy of Pediatrics (AAP) guidelines defined a positive UA for children between 2-24 months of age as a chemical dipstick test that is positive for either nitrites or leukocyte esterase (LE) or a microscopic analysis positive for either leukocytes or bacteria.[5] However, these AAP guidelines do not define threshold values for positive leukocytes, LE, or bacteria. For older children, what constitutes a positive UA is even less well defined. European guidelines published in 2015[6] and updated in 2021[7] also fail to give a specific definition for a positive UA. The 2021 guideline update, however, does define a negative UA as showing negative results for nitrites and LE on dipstick testing and no pyuria or bacteriuria on microscopic exam.
Given the lack of consensus definition for positive results, studies on pediatric UTI have used various thresholds for defining positive results. A urinalysis with a positive nitrite test is always considered positive.5,6,7,[8],[9],[10],[11],[12],[13] Positive LE values used range from “present”8,9,10,11 to greater than “trace.”12,13 The presence of bacteria is sometimes included in the definition of a positive UA,9 but not always.8,10,11,12,13 Positive white blood cell (WBC) values range from at least 5 WBCs per high powered field (HPF)10,13 to >10 WBC/HPF.9
This disparity between cutoffs used by various researchers has led to heterogeneity in how individual institutions and clinicians interpret pediatric UA results. Especially in UC settings, UAs are used as the initial diagnostic study to determine if empiric antibiotics or a urine culture are indicated.5,7 Adding greater clarity on appropriate threshold values for UAs would allow for more standardized practice in the diagnosis of UTI in children. Such standardization of thresholds could also improve antibiotic stewardship, as many children are treated empirically based on variable UA findings while the urine culture is pending.5,7
While there have been multiple studies evaluating appropriate diagnostic cutoffs to define a positive urine culture,[14],[15],[16] there has never been a study evaluating the appropriate cutoffs of WBCs, red blood cells (RBCs), LE, or nitrites on urine dipstick testing to warrant a positive UA in the pediatric UC setting.
In 2020, our system of pediatric UC centers undertook a large quality improvement (QI) project to improve the management of children with suspected UTI. The overview of this project has already been described in JUCM.[17] As part of this QI project, we reviewed 6,327 patient encounters that had corresponding UA values for these patients collected during the same visit.
We identified a lack of clarity on the operational definition of a “positive” UA in reviewing the results of this project. At the start of the project, we defined a UA as “positive” when either nitrites or at least moderate LE were present on dipstick testing, or at least 10 WBC/HPF or bacteria were present on the microscopic analysis. These definitions were informed by existing literature on the topic, but were somewhat arbitrary given the lack of a consensus definition of test positivity. To answer this question, the previously collected data was analyzed using a machine learning approach.
Machine learning, a branch of artificial intelligence, is dedicated to the creation of algorithms that enable computers to learn from data and make predictions or decisions. This technology is employed in a wide range of applications, including image and speech recognition, recommendation systems, decision trees, and predictive analytics.
Methods
This project was reviewed by the Eastern Virginia Medical School Institutional Review Board, which found that it met criteria for QI and was therefore granted “not human subjects research” status. Only deidentified data were analyzed as part of this project.
Urine samples were collected from 4 pediatric UC centers that were part of a single health system in Southeast Virginia between April 2018 and April 2020. Urinalyses and cultures were ordered at the discretion of the clinician. All patients with both a UA and urine culture from the same visit during the project period were included.
For urine samples from all reviewed charts LE, WBCs, nitrite, blood, and bacteria were analyzed as predictors for a positive urine culture. Clean catch and catheterized specimens were analyzed separately. For clean catch samples, cutoffs of both 50,000 and 10,000 colony forming units (CFUs) of a single or predominate organism were used as the definition of a positive urine culture. For catheterized samples, cutoffs of 10,000 and 50,000 CFUs were used.
For all samples, dipstick results were obtained using a CLINITEK Status+ Analyzer and Siemens Multistix 10 SG reagent sticks. Microscopic results were obtained from our affiliated clinical laboratory.
Prior to running classification models, univariate associations between each predictor and CFU cutoff were performed. Multivariate logistic regression was used to determine the UA findings that predict positive urine cultures. Twelve possible models for a positive UA were used as the basis of the analysis (Table 1). Some of these possible criteria were based on national guidelines. Because this was a QI project, other possible criteria were based on local treatment guidelines. The multicollinearity between predictors was evaluated using the variance inflation factor (VIF). The boosted C5.0 algorithm using the resampling method was the particular machine learning approach used in this case and was used to create decision trees. Boosted C5.0 is a well-known and widely use machine learning model that combines multiple classifiers to enhance predictive accuracy. Boosting improves model accuracy by leveraging misclassifications from the initial C5.0 decision tree models and adjusting to complex data relationships. In comparison to other decision tree models like C4.5 and CART, boosted C5.0 achieves superior accuracy with lower error rates and faster processing.
Model | Predictors |
---|---|
1. | LE+ nitrites+ WBCs+ blood+ bacteria |
2. | LE + Nitrites + WBCs5 + Blood + Bacteria |
3. | LE + Nitrites + WBCs10 + Blood + Bacteria |
4. | LE moderate + Nitrites + WBCs + Blood + Bacteria |
5. | LE moderate + Nitrites + WBCs5 + Blood + Bacteria |
6 | LE moderate + Nitrites + WBCs10 + Blood + Bacteria |
LE: leukocyte esterase (yes/no); Blood: negative vs positive; LE Moderate: moderate to large LE vs none or trace or small; Nitrites: negative vs positive; WBCs: white blood cells (yes/no); Bacteria: negative vs positive; WBCs5: WBCs at least 10-25; WBCs10: WBCs at least 10-20
Six combinations of predictors were then developed to create decision trees (Table 2). The performance of classification results was evaluated by sensitivity, specificity, accuracy, negative predictive value, F-measure, and area under curve (AUC).
The univariate associations between continuous variables and each respective CFU group were assessed using a t-test or Mann-Whitney test, while the correlations between categorical variables and each CFU group were assessed using chi-square or Fisher’s exact test. All statistical tests were performed using R Studio 4.1.0. All statistical tests were 2-sided, and p<0.05 was considered as statistically significant.
Results
A total of 5,075 clean catch specimens and 1,252 catheterized specimens were reviewed. During that time period, 158,981 patients were seen between all UC centers. Patients ranged in age from 14 days to 20 years. Eighteen percent of patients that were included in this data set had a history of a previous UTI, and most patients seen at our UC centers are healthy without a history of any type of urinary tract disease or anatomical abnormalities. For catheterized specimens, 74% of patients had a fever and 72% were under 2 years old. For clean catch specimens, 35% of patients had abdominal pain, 64% had urinary symptoms, and 22% had fever.
The results of the univariate model for catheterized specimens revealed significant correlation (P<0.001) between all possible predictors of a UTI, except for presence of RBCs, and a positive urine culture when using either 10,000 or 50,000 CFUs as the definition of a positive culture. The results of the univariate model for clean catch specimens revealed significant correlation (P<0.001) between all possible predictors of a UTI and a positive urine culture when using either 50,000 or 100,000 CFUs as the definition of a positive culture .
Table 3 presents the results of the logistic regression for each model for clean catch and catheterized specimens. The VIF criteria did not suggest any multicollinearity between independent variables in any model. For clean catch specimens, model 4, followed by model 9 had the highest AUC, suggesting that moderate LE, nitrites, WBCs≥5 or >10 and bacteria (all p<0.001) would be the strongest predictors of a positive urine culture. For catheterized specimens, model 3 and model 5 had the highest AUC, followed by models 1&2 and model 4, suggesting that LE, nitrites, and WBCs ≥5 (all p<0.001) would be the best factors for predicting a positive urine culture.
Model | 1&2 | 3 | 4 | 5 | 6 | 7 | 8 | 9 | 10 | 11 | 12 |
---|---|---|---|---|---|---|---|---|---|---|---|
Clean catch, CFUs 50,000 | |||||||||||
LE | <0.001 | <0.001 | – | <0.001 | – | – | – | – | – | – | – |
LE Moderate | – | – | <0.001 | – | <0.001 | <0.001 | – | <0.001 | – | <0.001 | – |
Blood | <0.001 | – | – | – | – | – | – | – | – | <0.001 | <0.001 |
WBCs | 0.50 | – | – | – | – | – | – | – | – | – | – |
WBCs5 | – | <0.001 | <0.001 | <0.001 | <0.001 | – | – | – | – | – | – |
WBCs10 | – | – | – | – | – | <0.001 | – | <0.001 | – | <0.001 | – |
Nitrites | <0.001 | <0.001 | <0.001 | <0.001 | <0.001 | <0.001 | <0.001 | <0.001 | <0.001 | <0.001 | <0.001 |
Bacteria | <0.001 | <0.001 | <0.001 | – | – | – | – | <0.001 | 0.001 | – | – |
LE&WBCs | – | – | – | – | – | – | <0.001 | – | <0.001 | – | <0.001 |
AUC | 0.8242 | 0.8430 | 0.8527 | 0.8349 | 0.8461 | 0.8365 | 0.7810 | 0.8482 | 0.8088 | 0.8454 | 0.7963 |
Clean catch, CFUs 100,000 | |||||||||||
LE | <0.001 | <0.001 | – | <0.001 | – | – | – | – | – | – | – |
LE Moderate | – | – | <0.001 | – | <0.001 | <0.001 | – | <0.001 | – | <0.001 | – |
Blood | <0.001 | – | – | – | – | – | – | – | – | <0.001 | 0.91 |
WBCs | 0.50 | – | – | – | – | – | – | – | – | – | – |
WBCs5 | – | <0.001 | <0.001 | <0.001 | <0.001 | – | – | – | – | – | – |
WBCs10 | – | – | – | – | – | <0.001 | – | <0.001 | – | <0.001 | – |
Nitrites | <0.001 | <0.001 | <0.001 | <0.001 | <0.001 | <0.001 | <0.001 | <0.001 | <0.001 | <0.001 | <0.001 |
Bacteria | <0.001 | <0.001 | <0.001 | – | – | – | – | <0.001 | <0.001 | – | – |
LE&WBCs | – | – | – | – | – | – | <0.001 | – | <0.001 | – | <0.001 |
AUC | 0.8376 | 0.8513 | 0.8605 | 0.8442 | 0.8539 | 0.8457 | 0.7993 | 0.8571 | 0.8215 | 0.8562 | 0.8143 |
Model | 1&2 | 3 | 4 | 5 | 6 | 7 | 8 | 9 | 10 | 11 | 12 |
Catheterized CFUs 10,000 | |||||||||||
LE | <0.001 | <0.001 | – | <0.001 | – | – | – | – | – | – | – |
LE Moderate | – | – | <0.001 | – | <0.001 | <0.001 | – | <0.001 | – | <0.001 | – |
Blood | 0.44 | – | – | – | – | – | – | – | – | 0.25 | 0.50 |
WBCs | 0.94 | – | – | – | – | – | – | – | – | – | – |
WBCs5 | – | <0.001 | <0.001 | <0.001 | <0.001 | – | – | – | – | – | – |
WBCs10 | – | – | – | – | – | <0.001 | – | <0.001 | – | <0.001 | – |
Nitrites | <0.001 | <0.001 | <0.001 | <0.001 | <0.001 | <0.001 | <0.001 | <0.001 | <0.001 | <0.001 | <0.001 |
Bacteria | 0.008 | 0.40 | 0.59 | – | – | – | – | 0.07 | 0.001 | – | – |
LE&WBCs | – | – | – | – | – | – | <0.001 | – | <0.001 | – | <0.001 |
AUC | 0.9223 | 0.9339 | 0.8980 | 0.9349 | 0.8961 | 0.8725 | 0.7865 | 0.8857 | 0.8297 | 0.8775 | 0.7974 |
Catheterized CFUs 50,000 | |||||||||||
LE | <0.001 | <0.001 | – | <0.001 | – | – | – | – | – | – | – |
LE Moderate | – | – | <0.001 | – | <0.001 | <0.001 | – | <0.001 | – | <0.001 | – |
Blood | 0.24 | – | – | – | – | – | – | – | – | 0.62 | 0.91 |
WBCs | 0.78 | – | – | – | – | – | – | – | – | – | – |
WBCs5 | – | <0.001 | <0.001 | <0.001 | <0.001 | – | – | – | – | – | – |
WBCs10 | – | – | – | – | – | <0.001 | – | <0.001 | – | <0.001 | – |
Nitrites | <0.001 | <0.001 | <0.001 | <0.001 | <0.001 | <0.001 | <0.001 | <0.001 | <0.001 | <0.001 | <0.001 |
Bacteria | 0.01 | 0.30 | 0.45 | – | – | – | – | 0.08 | 0.002 | – | – |
LE&WBCs | – | – | – | – | – | – | <0.001 | – | <0.001 | – | <0.001 |
AUC | 0.9237 | 0.9333 | 0.9037 | 0.9312 | 0.8991 | 0.8811 | 0.7992 | 0.8938 | 0.8380 | 0.8846 | 0.8078 |
AUC: area under the curve; CFU: colony forming units; LE: leukocyte esterase (yes/no); LE Moderate: moderate to large LE vs none or trace or small; WBCs: white blood cells (yes/no); WBCs5: WBCs negative or less than 5 vs. 5 or more; WBCs10: WBCs negative or less than 10 vs. 10 or more; Blood: negative vs positive; Nitrites: negative vs positive; Bacteria: negative vs positive; LE&WBCs: at least moderate LE and WBCs 10 or more
After logistic regression was performed, decision trees were developed using the previously described predictor combinations. The method for developing decision trees assessed the best combination of variables in each model and suggested the combination with the highest impact on the outcome. For models 4-6 WBCs, blood, and bacteria were not suggested as part of the best combination by this method. The best combination for these models was at least moderate LE and positive nitrites. When the non-ideal variables were dropped, these 3 models were identical and were therefore combined. For both clean catch and catheterized specimens, all of the best decision trees included a positive nitrite component.
Predictor | Accuracy | Error | Sensitivity | Specificity | F-measure | AUC | |
Model 1 | LE & Bacteria | 0.7168 | 0.2832 | 0.2518 | 0.7016 | 0.1940 | 0.7732 |
Model 2 | LE & WBCs5 | 0.7814 | 0.2186 | 0.3006 | 0.7795 | 0.2183 | 0.7884 |
Model 3 | LE & WBCs10 | 0.8473 | 0.1527 | 0.3744 | 0.8705 | 0.2379 | 0.7613 |
Model 4-6 | LE moderate | 0.8714 | 0.1286 | 0.3992 | 0.9253 | 0.2041 | 0.6714 |
Predictor | Accuracy | Error | Sensitivity | Specificity | F-measure | AUC | |
Model 1 | LE & Bacteria & Blood | 0.7647 | 0.2352 | 0.2345 | 0.7615 | 0.1814 | 0.7810 |
Model 2 | LE & WBCs5 | 0.7723 | 0.2277 | 0.2444 | 0.7677 | 0.1884 | 0.7955 |
Model 3 | LE & WBCs10 | 0.8454 | 0.1546 | 0.3082 | 0.8603 | 0.2123 | 0.7711 |
Model 4-6 | LE moderate | 0.8775 | 0.1225 | 0.3251 | 0.9182 | 0.1855 | 0.6720 |
Predictor | Accuracy | Error | Sensitivity | Specificity | F-measure | AUC | |
Model 1 | LE | 0.9241 | 0.0759 | 0.6424 | 0.9388 | 0.3594 | 0.8771 |
Model 2 | LE & WBCs5 | 0.9342 | 0.0658 | 0.7520 | 0.9703 | 0.3534 | 0.8185 |
Model 3 | LE & WBCs10 | 0.9325 | 0.0674 | 0.8506 | 0.9876 | 0.3246 | 0.7562 |
Model 4-6 | LE moderate | 0.9309 | 0.0691 | 0.8933 | 0.9923 | 0.3102 | 0.7338 |
Predictor | Accuracy | Error | Sensitivity | Specificity | F-measure | AUC | |
Model 1 | LE | 0.9182 | 0.0818 | 0.5698 | 0.9276 | 0.3389 | 0.8818 |
Model 2 | LE & WBCs5 | 0.9334 | 0.0667 | 0.6720 | 0.9615 | 0.3401 | 0.8250 |
Model 3 | LE & WBCs10 | 0.9351 | 0.0649 | 0.7586 | 0.9803 | 0.3158 | 0.7606 |
Model 4-6 | LE moderate | 0.9401 | 0.0599 | 0.8400 | 0.9887 | 0.3198 | 0.7526 |
AUC: area under the curve; CFU: colony forming units; LE: leukocyte esterase (yes/no); LE Moderate: moderate to large LE vs none or trace or small; WBCs: white blood cells (yes/no); WBCs5: WBCs negative or less than 5 vs. 5 or more; WBCs10: WBCs negative or less than 10 vs. 10 or more; Blood: negative vs positive; Nitrites: negative vs positive; Bacteria: negative vs positive; LE&WBCs: at least moderate LE and WBCs 10 or more
Because it is already known that a positive nitrite value on UA is strongly correlated with a positive urine culture, a separate analysis of nitrite negative specimens was performed (Table 4). For nitrite negative catheterized specimens, LE present, and WBCs greater than or equal to 5 had the best accuracy and F-measure for both CFU cutoffs. This was followed by LE greater than or equal to moderate. For clean catch specimens, LE greater than or equal to moderate had the best accuracy and F-measure for both CFU cutoffs. This was followed by WBCs ≥10 and LE present. Figures 1 and 2 show the decision trees for the best predictive models for nitrite negative clean catch and catheterized samples.
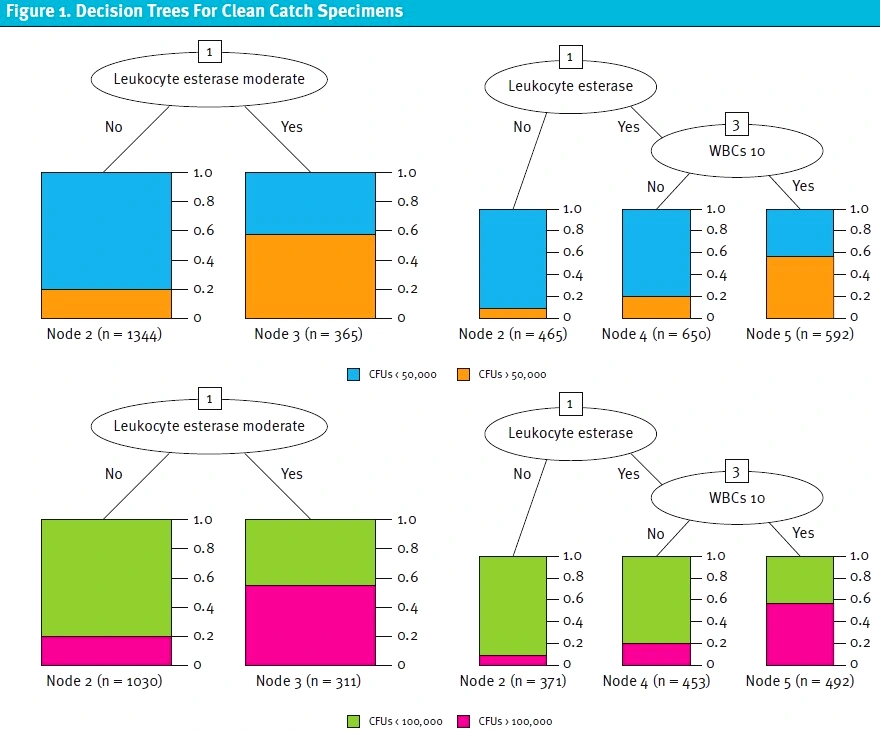
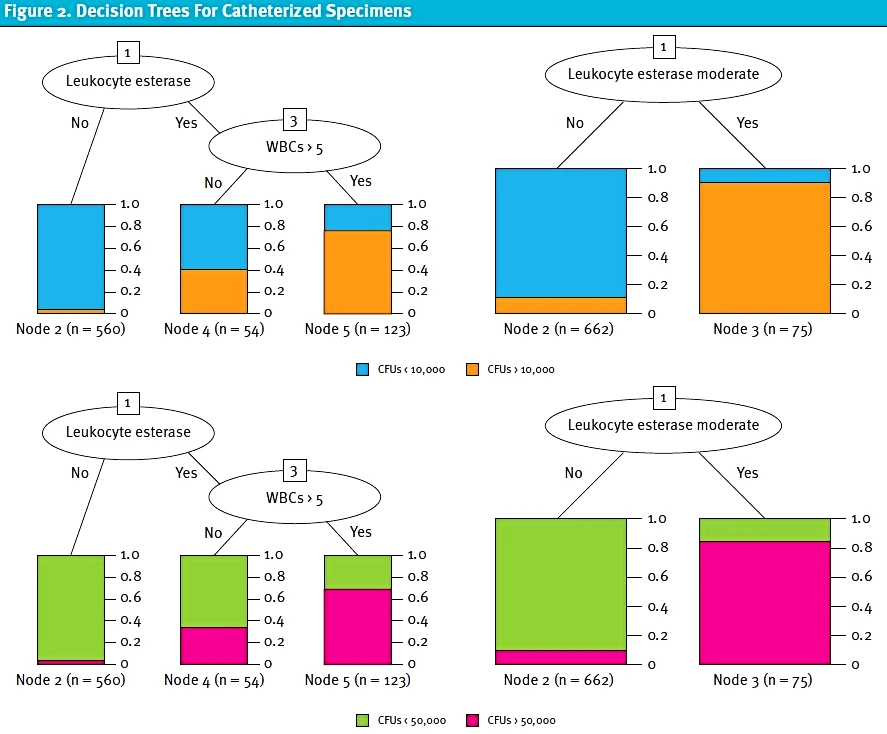
Discussion
These results indicate that the best predictive models for a positive UA include positive nitrites and specific LE and WBC cutoffs. The most predictive UA cutoffs differed based off collection method, which is consistent with existing knowledge about the significance of varying amounts of WBCs and bacteria in catheterized versus bag collection. To our knowledge, this is the first study to evaluate specific thresholds for a positive UA using a machine learning approach. Commonly used cutoffs cited in other studies include >5 WBCs/HPF or presence of any LE or nitrites, but these had not been statistically evaluated for appropriateness and are often used universally regardless of collection method.5,7,8,[18],[19]
The presence of nitrites, which current guidelines deem to indicate a “positive” urinalysis7-13 was corroborated as a strong predictor of a positive urine culture (p <0.001). Excluding positive nitrites, moderate LE was found to have a high predictivity for both catheterized and clean catch samples (accuracy = 0.9401 and 0.8775, respectively). Presence of LE and WBCs >5 was also predictive of a positive urine culture in catheterized samples (accuracy = 0.9342, F-measure = 0.3534), while presence of LE and WBCs >10 was also predictive of positive urine culture in catheterized samples (accuracy = 0.8454, F-measure = 0.2123). All of our predictors were noted to have a higher accuracy in catheterized samples versus clean catch samples (Table 4).
Limitations
This project had several limitations. We used a specific colorometric urine dipstick. Other dipsticks may have different semi-qualitative (eg, trace, moderate, etc.) results for corresponding quantities of LE, blood, protein etc. Likewise, the number of WBC, RBC, and bacteria in a given sample is somewhat subjectively determined by a laboratory technician. We examined only catheterized and clean catch samples and did not evaluate cutoffs for suprapubic aspiration or sterile urine bags. However, using similar methodology, this could be evaluated in a future study. This was an observational study, and urine samples and cultures were at the discretion of the treating clinician and disassociated from the patients. This limits assessment of patient factors that may influence these thresholds.
It is unclear how encounters and patients with complete UA and culture data may be different from those in which one or both of these studies were not performed. These analyses were part of a local quality improvement project at a single system of pediatric UC centers, and generalizability could be verified if the results were to be replicated in other geographies and care settings. Additionally, some UC centers do not have access to rapid urine microscopy results and/or materials or staff required to obtain catheterized urine specimens in children.
While this study was limited to a single group of UC centers, it did contain a large number of mostly healthy patients. Most catheterized specimens were obtained in children under the age of 2 years presenting with fever, while clean catch samples were commonly obtained from patients presenting with fever, urinary symptoms, or abdominal pain. Our results would therefore most likely be applicable to other pediatric UC centers that see primarily healthy children with common complaints that could be concerning for a UTI.
Future studies should investigate the clinical application of thresholds prospectively to evaluate their effects on treatment decisions and patient outcomes. We do not suggest that these criteria for positivity of UA be adopted without further research in guiding decisions around urine culture or empiric treatment. Urine cultures provide critical information to help guide UTI treatment and assure antibiotic stewardship through pathogen identification and susceptibility analysis.5,7 These new criteria could, however, improve urine culture stewardship and, by extension, antibiotic stewardship. This could decrease cost by eliminating unnecessary urine cultures and antibiotic courses in the event of a negative UA, as well as reduce the negative effects of unnecessary antibiotic use such as the colonization of resistant bacteria, Clostridium difficile infection, and potential drug adverse effects.[20],[21]
Finally, it is noteworthy that urine concentration (ie, specific gravity) has been shown to affect thresholds of pyuria which predict a positive urine culture in pediatric emergency department patients.[22] Specific gravity (SG) was not included in our regression model and therefore future studies could clarify appropriate cut-off values for pediatric UAs for various ranges of SG.
Conclusion
The secondary analysis of data from our QI project using machine learning to evaluate pediatric urinalyses indicated that specific value cutoffs for LE, nitrites, bacteria, and RBC could predict the likelihood of a positive urine culture in clean catch and catheterized samples. More data is needed to determine if this holds true for different collection methods.
Manuscript submitted May 17, 2024, accepted September 23, 2024.
Author Affiliations: Kari Flicker, BS, Eastern Virginia Medical School. Jessica Parrott, DNP, CPNP-PC, CNE, Children’s Hospital of the King’s Daughters Urgent Care, Norfolk, Virginia; Old Dominion University. Tammy Speerhas, DNP, FNP-C, Children’s Hospital of the King’s Daughters Urgent Care, Norfolk, Virginia; Old Dominion University. Turaj Vazifedan, DHSc, King’s Daughters Urgent Care, Norfolk, Virginia; Eastern Virginia Medical School. Theresa Guins, MD, Children’s Hospital of the King’s Daughters Urgent Care, Norfolk, Virginia; Eastern Virginia Medical School. Jeffrey Bobrowitz, MD, Children’s Hospital of the King’s Daughters Urgent Care, Norfolk, Virginia; Eastern Virginia Medical School. Anne McEvoy, MD, Northwell Health. Jade Eves, PA-C, Children’s Hospital of the King’s Daughters Urgent Care, Norfolk, Virginia; Eastern Virginia Medical School. Debra Conrad, MD, retired, King’s Daughters Urgent Care, Norfolk, Virginia. Benjamin Klick, MD, Children’s Hospital of the King’s Daughters Urgent Care, Norfolk, Virginia; Eastern Virginia Medical School.
References
- [1]. Jakobsson B, Esbjörner E, Hansson S. Minimum incidence and diagnostic rate of first urinary tract infection. Pediatrics. 1999;104(2 Pt 1):222-226.
- [2]. Mårild S, Jodal U. Incidence rate of first-time symptomatic urinary tract infection in children under 6 years of age. Acta Paediatr. 1998;87(5):549-552.
- [3]. Echeverry G, Hortin GL, Rai AJ. Introduction to urinalysis: historical perspectives and clinical application. Methods Mol Biol. 2010;641:1-12.
- [4]. Simerville JA, Maxted WC, Pahira JJ. Urinalysis: a comprehensive review [published correction appears in Am Fam Physician. 2006 Oct 1;74(7):1096]. Am Fam Physician. 2005;71(6):1153-1162.
- [5]. Roberts KB. Urinary tract infection: clinical practice guideline for the diagnosis and management of the initial UTI in febrile infants and children 2 to 24 months. Pediatrics. 2011;128(3):595-610.
- [6]. Stein R, Dogan HS, Hoebeke P, et al. Urinary tract infections in children: EAU/ESPU guidelines. Eur Urol. 2015;67(3):546-558. 1825
- [7]. ‘t Hoen LA, Bogaert G, Radmayr C, et al. Update of the EAU/ESPU guidelines on urinary tract infections in children. J Pediatr Urol. 2021;17(2):200-207
- [8]. Tzimenatos L, Mahajan P, Dayan PS, et al. Accuracy of the Urinalysis for Urinary Tract Infections in Febrile Infants 60 Days and Younger. Pediatrics. 2018;141(2).
- [9]. Glissmeyer EW, Korgenski EK, Wilkes J, et al. Dipstick screening for urinary tract infection in febrile infants. Pediatrics. 2014;133(5):e1121-1127.
- [10]. Zorc JJ, Levine DA, Platt SL, et al. Clinical and demographic factors associated with urinary tract infection in young febrile infants. Pediatrics. 2005;116(3):644-648.
- [11]. Dayan PS, Bennett J, Best R, et al. Test characteristics of the urine Gram stain in infants <or= 60 days of age with fever. Pediatr Emerg Care. 2002;18(1):12-14.
- [12]. McGillivray D, Mok E, Mulrooney E, Kramer MS. A head-to-head comparison: “clean-void” bag versus catheter urinalysis in the diagnosis of urinary tract infection in young children. J Pediatr. 2005;147(4):451-456.
- [13]. Bachur R, Harper MB. Reliability of the urinalysis for predicting urinary tract infections in young febrile children. Arch Pediatr Adolesc Med. 2001;155(1):60-65.
- [14]. Swerkersson S, Jodal U, Åhrén C, Sixt R, Stokland E, Hansson S. Urinary tract infection in infants: the significance of low bacterial count. Pediatr Nephrol. 2016;31(2):239-245.
- [15]. Primack W, Bukowski T, Sutherland R, Gravens-Mueller L, Carpenter M. What Urinary Colony Count Indicates a Urinary Tract Infection in Children? J Pediatr. 2017;191:259-261.e251.
- [16]. Tullus K. Low urinary bacterial counts: do they count? Pediatr Nephrol. 2016;31(2):171-174.
- [17]. Klick B, Speerhas T, Parrott J, et al. A quality improvement project to improve management of urinary tract infections in a system of pediatric urgent care centers. J Urgent Care Med. 2023;17(9):23-29.
- [18]. Schmidt B, Copp HL. Work-up of Pediatric Urinary Tract Infection. Urol Clin North Am. 2015;42(4):519-526.
- [19]. American Academy of Pediatrics. Practice parameter: the diagnosis, treatment, and evaluation of the initial urinary tract infection in febrile infants and young children. Committee on Quality Improvement. Subcommittee on Urinary Tract Infection. Pediatrics. 1999;103(4 Pt 1):843-852.
- [20]. Ge IY, Fevrier HB, Conell C, et al. Reducing risk of Clostridium difficile infection and overall use of antibiotic in the outpatient treatment of urinary tract infection. Ther Adv Urol. 2018;10(10):283-293.
- [21]. Pietrucha-Dilanchian P, Hooton TM. Diagnosis, Treatment, and Prevention of Urinary Tract Infection. Microbiol Spectr. 2016;4(6).
- [22]. Chaudhari PP, Monuteaux MC, Shah P, Bachur RG. The Importance of Urine Concentration on the Diagnostic Performance of the Urinalysis for Pediatric Urinary Tract Infection. Ann Emerg Med. 2017;70(1):63-71.e8. doi:10.1016/j.annemergmed.2016.11.042
Download the Article PDF: Development of a Positive Urinalysis Criteria Using a Machine Learning Approach